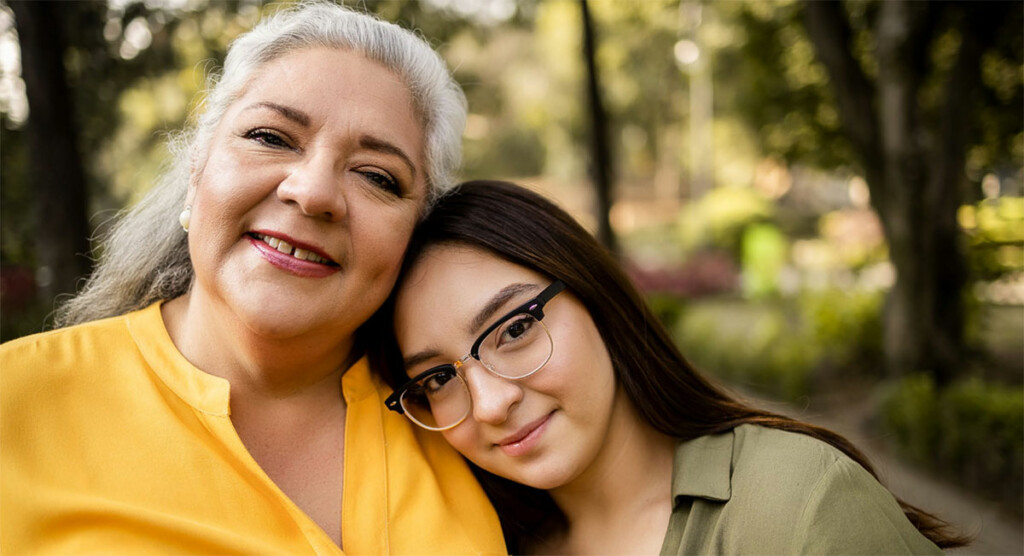
OCRA Sponsors NCCN 2025 Guidelines for Cervical Cancer Patients
This article was updated in May 2025 to reflect NCCN’s release of the 2025 Guidelines for Cervical Cancer Patients. OCRA is a proud sponsor of 2025 Cervical Cancer Updates. We … Continued