A recent study published in Nature Cancer talks about harnessing the power of machine learning to help predict prognosis and response to treatment for those with high-grade serous ovarian cancer (HGSOC).
The study is co-authored by OCRA grantees Sohrab Shah, PhD, of Memorial Sloan Kettering Cancer Center – a two-time OCRA grant recipient, most recently of OCRA’s Collaborative Research Development Grant in collaboration with Microsoft AI for Health – and Dmitriy Zamarin, MD, PhD, of Memorial Sloan Kettering Cancer Center, along with research teams at MSKCC, Weill Cornell Medicine and Columbia University.
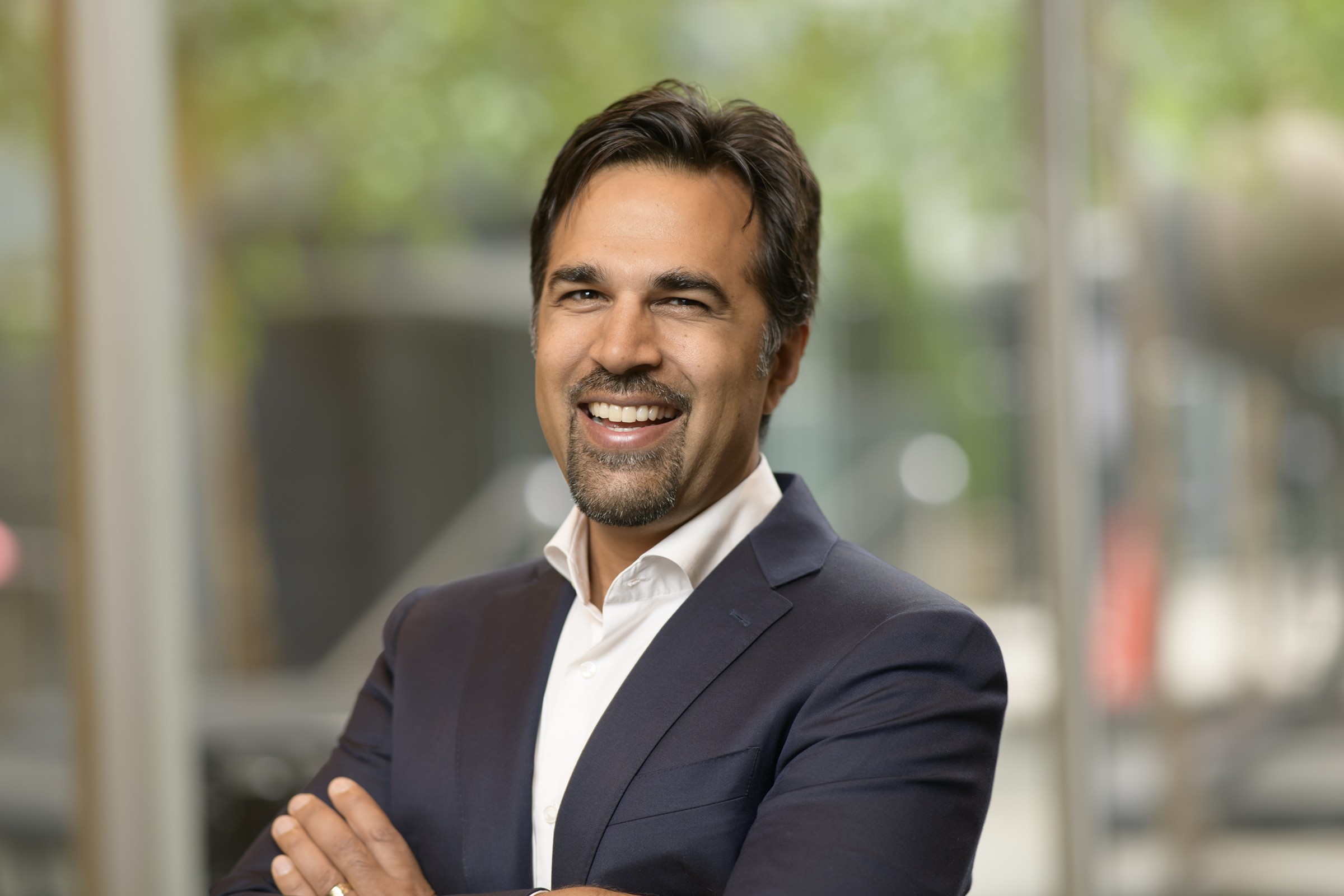
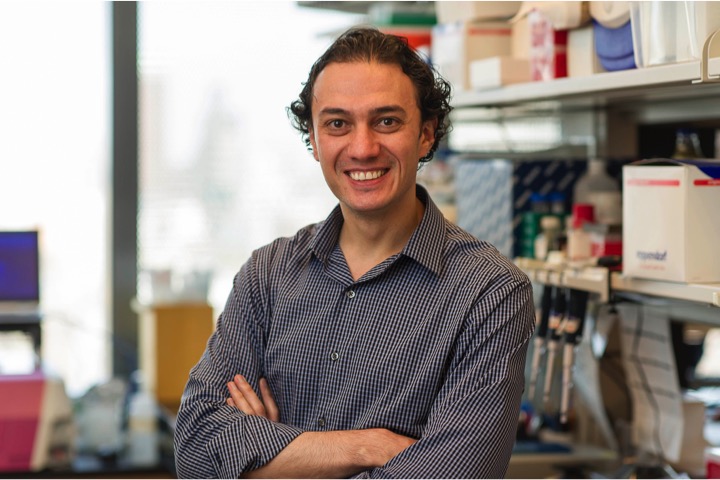
HGSOC is the most common subtype of ovarian cancer, yet response to treatment can be unpredictable and poses significant challenges. Several clinical factors are known to affect prognosis, including patient age, stage of disease, and amount of residual disease after debulking surgery is completed.
In addition, molecular and genomic factors gathered through imaging tests such as CT and radiological scans, and histological specimens of individual cancer cells, can provide useful information for predicting prognosis and response to treatment.
These factors all have a role in an individual’s predicted outcome, but until recently, the ovarian cancer field has lacked a way to combine disparate data and analyze it to discover overarching patterns. A holistic picture of this data, specifically around treatment effectiveness and resistance, could aid gynecologic oncologists in predicting how patients may respond to standard treatment; and aid in selecting primary treatment, planning surveillance and maintenance therapy, as well as considering clinical trials.
The study’s authors set out to bridge the gap between disparate data sets and gain potentially actionable insights toward finding more effective treatments for individual patients. Researchers analyzed data from 444 patients with HGSOC, including 296 patients treated at MSKCC and 148 patients from The Cancer Genome Atlas Ovarian Cancer (TGCA-OV) data. The cohort included patients with varying stages of disease and treatment histories, with the majority of patients having stage III or stage IV ovarian cancer.
Researchers separated the cohort into a training group and a test group, and analyzed genomic, histopathological, radiological and clinical data, all obtained during their individual routine diagnostic workups. Participants in the test group had full data available for analysis, while those in the training group had varying amounts, with the largest difference being a lack of clinical data around treatment regimens in the TGCA-OV set.
To gain insights around treatment resistance, particular attention was paid to homologous recombination-deficient (HRD) disease status, presence of BRCA1 or BRCA2 mutations, and associated response to platinum-based chemotherapy and PARP inhibitors.
Results of the study revealed correlations between average tumor nuclear size and presentation of omental implants (ovarian cancer tumor cells found in the omentum, a layer of fatty tissue in the peritoneal cavity), as picked up in imaging tests, to be significant prognostic factors. The study’s authors believe that this represents the first investigation of prognostic information captured within omental models, and view this as a promising new avenue for study because of its ubiquity in advanced-stage disease, as well as its relative ease of segmentation.
Researchers found that the study’s RI (radiological and histopathological) and GRH (genomic, radiological and histopathological) models provided the most significant results and held particular promise. The full combined genomic, histopathological, radiological and clinical (GHRC) model’s results were more disparate, in part because of the relatively small test size, and because clinical data on a significant segment of the study cohort was limited.
The study’s authors have made data from the MSKCC patient cohort available for analysis from the wider scientific community, with the hope that this data will contribute to future discoveries.
OCRA profiled co-authors of this exciting study in our Meet a Scientist series. Read interviews with Dr. Sohrab Shah and Dr. Dmitriy Zamarin to learn more about their individual ovarian cancer research projects, and what they each hope to achieve for future generations.