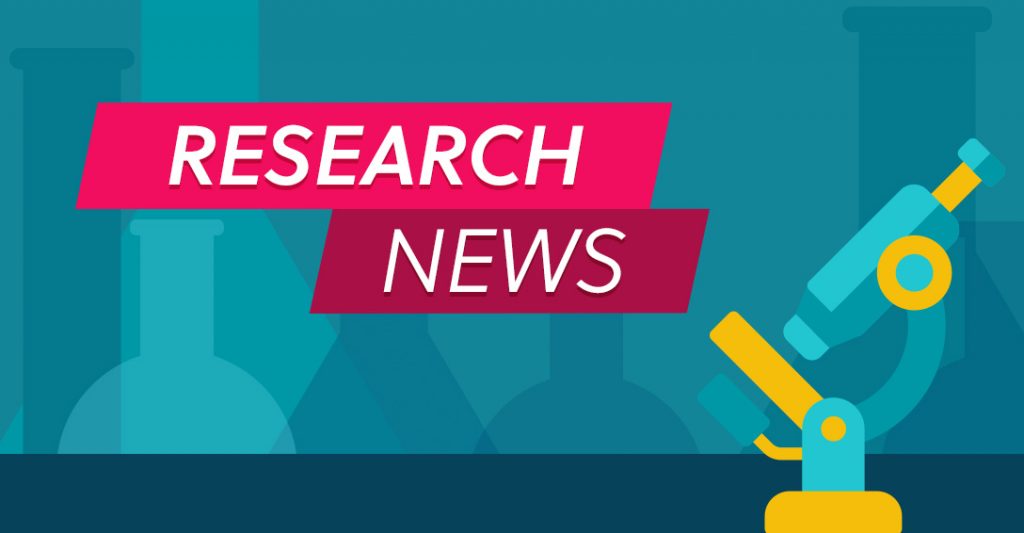
Recent OCRA-funded research has resulted in the creation of a new bioinformatics tool that holds potential for predicting the best treatment combinations for cancer patients.
Bioinformatics is a scientific discipline that enables researchers to collect, process and analyze a vast amount of biological and medical data via computers and statistical techniques. In the case of this recently developed bioinformatics platform, known as REcurrent Features LEveraged for Combination Therapy (REFLECT), scientists at The University of Texas MD Anderson Cancer Center have integrated machine learning and cancer informatics algorithms to develop a comprehensive resource of precision combination therapies. Their aim is to improve the efficacy of targeted therapies in treating cancer.
“Our ultimate goal is to make precision oncology more effective and create meaningful patient benefit,” said principal investigator Anil Korkut, PhD, assistant professor of Bioinformatics and Computational Biology at MD Anderson. He presented his team’s findings at the American Association for Cancer Research (AACR) Annual Meeting 2022. The research results were also published in Cancer Discovery.
“We believe REFLECT may be one of the tools that can help overcome some of the current challenges in the field by facilitating both the discovery and the selection of combination therapies matched to the molecular composition of tumors,” Dr. Korkut explained.
Targeted therapies, such as PARP inhibitors, are treatments that identify and attack certain molecules in cancer cells while doing minimal harm to normal cells. Though targeted therapies have been successful in improving outcomes for some cancer patients, tumor resistance to these anticancer treatments continues to be a major challenge.
According to Dr. Korkut and his team, one reason for this limited success is that monotherapies against a single target are typically used, which can often lead to treatment resistance. As Dr. Korkut notes, tumor growth is typically promoted by “co-occurring molecular aberrations,” which are abnormalities that exist simultaneously and drive the progression of malignancies (one example is the occurrence of genetic mutations in two signaling pathways). As such, identifying and targeting both aberrations at the same time via precision combination therapies may prove to be more effective at combatting tumor resistance than using monotherapies against a single target.
The researchers drew on core principles in cancer genomics and therapeutics when creating the REFLECT tool, including the aforementioned idea that tumor progression is driven by two or more alterations occurring at the same time. They designed the platform to analyze biological tumor features (such as genetic mutations and aberrations in protein expression) and identify frequent co-occurring abnormalities that could be targeted by multiple therapies.
After building REFLECT, Dr. Korkut and his team analyzed pan-cancer datasets representing more than 10,000 patients and 33 cancer types. This information, which they pulled from both MD Anderson and publicly available sources, generated a total of 201 patient cohorts, with each cohort defined by a single biomarker that could be targeted by therapy, such as an EGFR mutation or an overexpression of the PD-L1 protein.
Within each cohort, the researchers used REFLECT to find additional alterations that could also potentially serve as therapeutic targets, thus creating sub-cohorts that might benefit from specific combination therapies. Across all cohorts, the researchers found a total of 2,166 drug combinations that could be matched to the co-occurring alterations that were identified.
“In total, 45% of the patients in the meta-cohort were matched to at least one combination therapy,” according to the researchers.
In order to validate the REFLECT approach, Dr. Korkut and his team conducted a retrospective analysis of publicly available pre-clinical and clinical studies to compare drug combinations used in those trials. They looked at the combinations matched by REFLECT versus the combinations not matched by REFLECT. In pre-clinical trials that used PDX models (cancer models in which human tumor tissue is implanted into a mouse for research purposes), REFLECT-matched combinations had a 34.5% decrease in median tumor volume. Comparatively, combinations not matched by the REFLECT tool had a 5.1% increase in median tumor volume.
“While REFLECT is still a concept that requires additional validation, we anticipate a great opportunity to translate this work into real clinical benefits,” Dr. Korkut said.